Even if you use ChatGPT “just for research,” it’s still dangerous
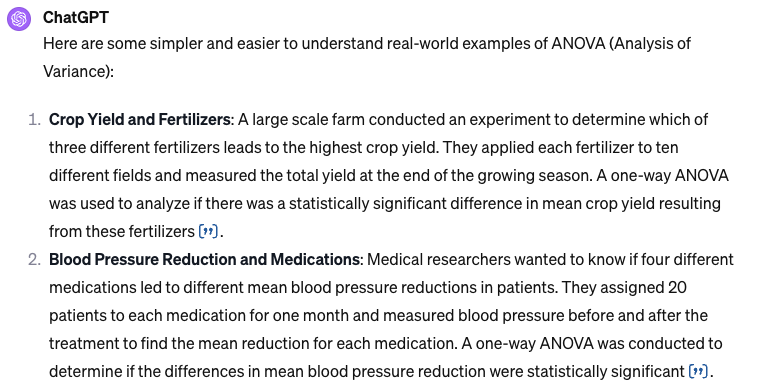
I’d never use ChatGPT to write for me: it’s both boring and unreliable. But that said, it’s still quite useful, especially as a replacement for search (I’m using ChatGPT-4, which integrates with Microsoft Bing including up-to-date content.)
The research method is simple: ask ChatGPT to find things, then check the source it finds to see if it is useful, trustworthy, and accurate. It’s much more effective than Google Search, especially when searching for concepts rather than specific details.
But as I learned recently, there are still plenty of unsuspected pitfalls.
How ChatGPT deluded itself and almost, me
I was searching for representative examples of the statistical concept “analysis of variance” in a legitimate study. Once I found them, I’d use them in a piece I’m writing for a client. I already know how analysis of variance, often called “ANOVA,” works and why it is useful. I just want to show an example of how it was used in a real-world situation.
Here was my first prompt:
Give an excellent real-world example of an analysis of variance that shows variance from several factors.
ChatGPT’s response was not a real-world example, it was a hypothetical:
Analysis of variance (ANOVA) is a powerful statistical technique used to determine if there are any statistically significant differences between the means of three or more independent (unrelated) groups. Here’s an excellent real-world example that demonstrates how ANOVA can be applied to understand variance from several factors:
Example: Impact of Diet and Exercise on Weight Loss
Context: A health research institute wants to understand the effectiveness of different weight loss strategies. They consider two main factors: diet and exercise. They design an experiment with . . . [continues in this vein]
If you were trying to understand how analysis of variance works, this would be helpful. But I didn’t need a made-up tutorial example. So I followed up with this:
I want a link to a real-world study, not a hypothetical like this one.
The result was an actual study, but one that was too convoluted and complex for me to use as an example. So my next prompt was this:
I want something simpler and easier to understand
ChatGPT responded with three examples. The quote marks in the brackets were links to web pages.
Here are some simpler and easier to understand real-world examples of ANOVA (Analysis of Variance):
- Crop Yield and Fertilizers: A large scale farm conducted an experiment to determine which of three different fertilizers leads to the highest crop yield. They applied each fertilizer to ten different fields and measured the total yield at the end of the growing season. A one-way ANOVA was used to analyze if there was a statistically significant difference in mean crop yield resulting from these fertilizers. [“]
- Blood Pressure Reduction and Medications: Medical researchers wanted to know if four different medications led to different mean blood pressure reductions in patients. They assigned 20 patients to each medication for one month and measured blood pressure before and after the treatment to find the mean reduction for each medication. A one-way ANOVA was conducted to determine if the differences in mean blood pressure reduction were statistically significant. [“]
- [list continues with two more examples]
This looked promising. So I clicked on the links . . . and was once again disappointed.
The first example was from an article on a site called statology, “4 examples of using ANOVA in real life.” The article was a good basic explanation with four illustrative hypothetical examples. The links all went to the same article: the first was about crop yield, the second about blood pressure, and the other two exactly matched what ChatGPT told me about.
Like any lazy researcher, ChatGPT had confused examples on a site that used the world “real-life” a bunch of times with actual, real-life examples.
This is just the sort of result you’d get in a Google or Bing search for “ANOVA” and “real-life.” But in this case, the answer appears far more credible since it’s a textual response that appears to fit the query.
Glib. But wrong.
Never trust an AI
This kind of convincing but wrong answer is exactly the type of pitfall AI generates.
It’s a great research tool. But never trust it. Because while it “knows” everything, it understands nothing.
The more I learn about generative technologies, the clearer my criticism becomes. Even with prompts beforehand and editorial judgment afterward, the difference between human and machine writing remains.
Writers use words to convey ideas; AI text generators use words.